In recent years, healthcare has been undergoing a profound transformation with the integration of cutting-edge technologies, and one of the most remarkable innovations is predictive analytics. Predictive analytics leverages the power of data, artificial intelligence, and machine learning to foresee medical events, enhance patient care, optimize resource management, and reduce costs. In this guide, we will review the pivotal role of predictive analytics in healthcare, highlighting its role and application in Remote Patient Monitoring (RPM).
What is Predictive Analytics Healthcare?
Predictive analytics in healthcare involves the analysis of historical and real-time data to predict future outcomes, enabling healthcare professionals to make informed decisions and take proactive measures. It employs algorithms to identify patterns, trends, and anomalies in data, allowing for the forecasting of health-related events such as disease outbreaks, patient readmissions, and clinical deterioration.
Predictive Analytics: Applications in Healthcare
Disease Prevention and Early Intervention: Predictive analytics can assist in identifying high-risk individuals for specific diseases by analyzing patient data and family histories. It helps in early diagnosis, enabling medical professionals to intervene before a condition worsens.
Improved Patient Care: By predicting patient deterioration, healthcare providers can prioritize their attention to patients who are at higher risk. This ensures timely interventions, reducing adverse events and hospital readmissions.
Resource Optimization: Hospitals and healthcare providers can use predictive analytics to optimize resource allocation, ensuring that staff, equipment, and facilities are efficiently utilized, reducing operational costs.
Drug Development: Pharmaceutical companies use predictive analytics to expedite drug development. This technology analyzes biological data to predict how drugs might react in clinical trials.
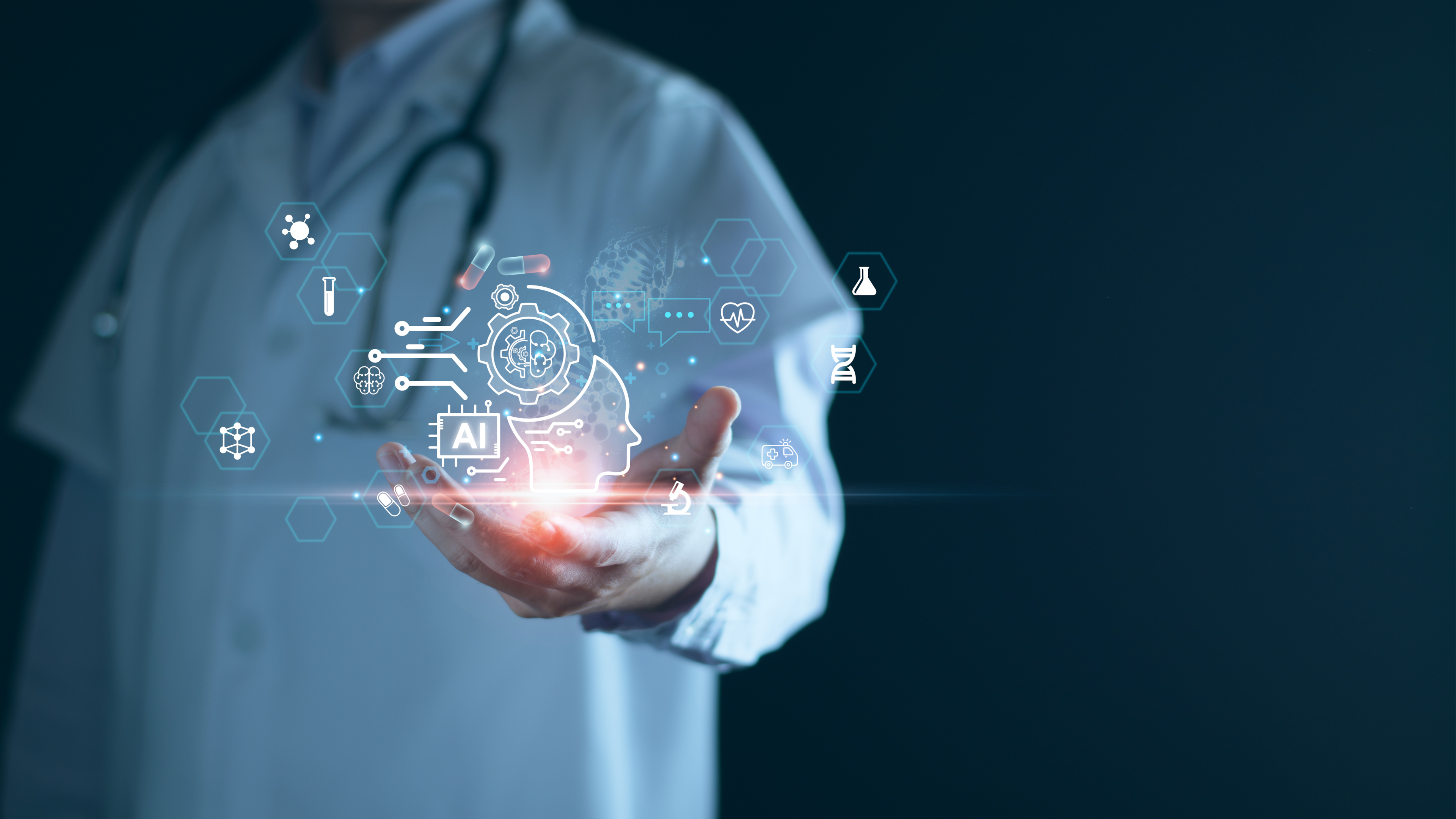
How Does Predictive Analytics Apply to Remote Patient Monitoring (RPM) Programs?
Predictive analytics plays a key role in enhancing the effectiveness of Remote Patient Monitoring (RPM) through the analysis of near real-time patient data collected by cellular-enabled devices. RPM involves the use of wearable sensors and connected devices to continuously monitor a patient’s health, sending data to a monitoring platform accessible to healthcare providers for monitoring, analysis, intervention.
RPM and Early Detection of Health Deterioration Using Predictive Models
Remote Patient Monitoring (RPM) programs utilize cellular-enabled devices to collect and transmit a patient’s health data to healthcare providers, creating a continuous feedback loop that allows for early intervention. These devices, ranging from wearable sensors to smart home monitors, capture vital signs, activity levels, medication adherence, and more. The data is then sent to a secure platform accessible to medical professionals.
Predictive analytics plays a pivotal role in early detection. By leveraging machine learning and data analysis techniques, healthcare providers can identify patterns and trends in a patient’s health data. These predictive models continuously process vast amounts of information, including historical patient data, to foresee potential health issues. This proactive approach helps in several ways:
Identification of At-Risk Patients: Predictive models can identify patients at higher risk for specific health deteriorations. For example, in chronic diseases like diabetes or heart failure, algorithms can predict exacerbations based on historical healthcare data and near real-time readings.
Near Real-Time Alerts: Customized thresholds are set for each patient. When readings deviate from their baseline or cross predetermined limits, healthcare providers receive near real-time alerts. This ensures that subtle health changes are not overlooked.
Cost Savings: By intervening early, the overall healthcare industry and organizations can reduce healthcare costs. Preventing hospitalizations, severe health events, and emergency department visits not only saves money but also improves patient quality of life.
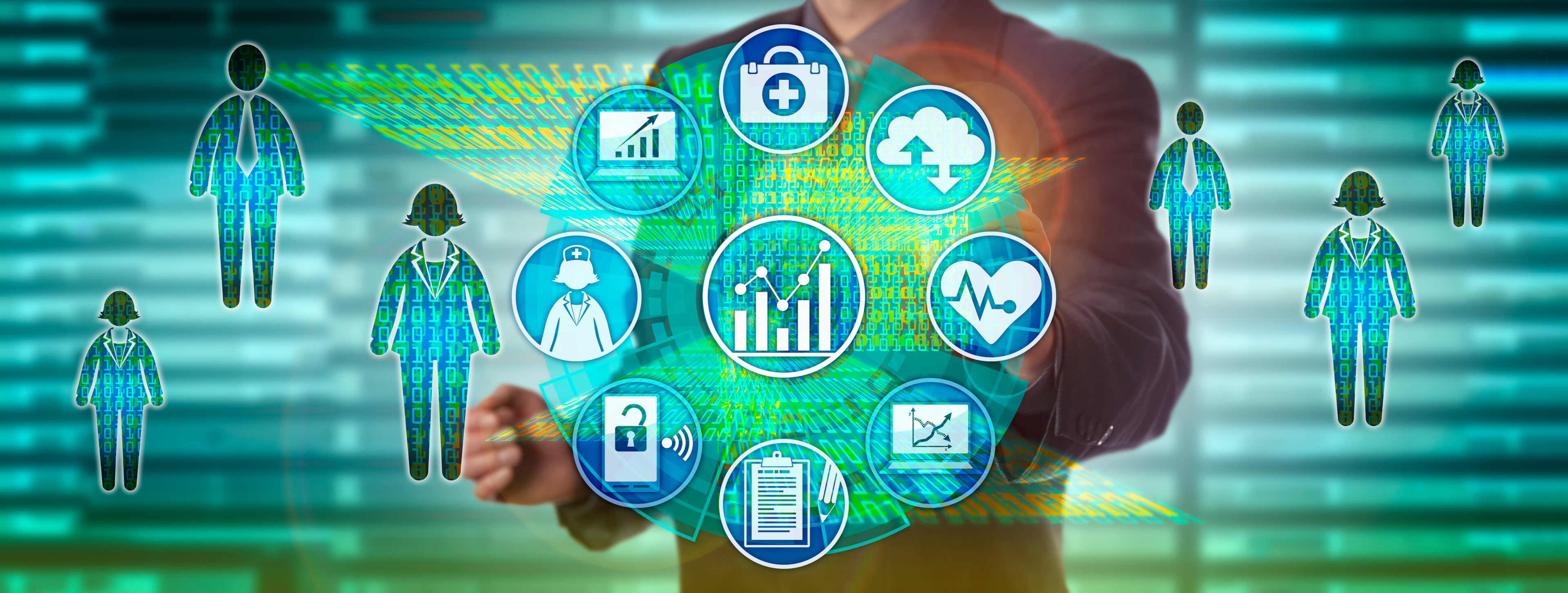
Real-Life Scenarios
Consider a patient with congestive heart failure. RPM devices continuously monitor their weight, blood pressure, and heart rate. Predictive analytics algorithms analyze this data, looking for signs such as sudden weight gain or elevated blood pressure. When the algorithms identify trends that indicate potential fluid retention or cardiac stress, they alert the healthcare team. This early alert allows the healthcare provider to make timely adjustments to the patient’s medication or treatment plan, potentially preventing a severe exacerbation.
In the case of chronic health conditions, like chronic obstructive pulmonary disease (COPD) or hypertension, early detection can lead to effective intervention and the prevention of complications that might otherwise result in emergency room visits or hospitalizations.
Personalized Care Plans for RPM Patients Using Predictive Analytics
In the evolving healthcare systems, personalized care plans have become a cornerstone of improving patient outcomes and enhancing the efficiency of care delivery. When it comes to Remote Patient Monitoring (RPM), the integration of personalized care plans with predictive analytics offers a revolutionary approach to patient-centered healthcare.
This synergy ensures that each patient receives a customized and proactive approach to managing their health, ultimately leading to better results. Personalized care plans not only improve patient outcomes but also lead to cost savings by reducing unnecessary hospitalizations or emergency room visits.
Implementing Personalized Care Plans in RPM
To implement personalized care plans for RPM patients, healthcare providers follow these steps:
Data Collection: RPM devices collect near real-time patient data, such as vital signs, symptoms, and medication adherence. This data is continuously transmitted to a secure platform.
Data Integration: Predictive analytics models integrate RPM data with the patient’s electronic health records, historical health data, and other relevant information.
Risk Assessment: The predictive model calculates the patient’s risk score based on data analysis. The patient’s unique risk factors and potential health issues are identified.
Plan Development: Healthcare providers, including physicians, nurses, and care teams, collaborate to develop personalized care plans. These plans consider the patient’s risk profile and may involve lifestyle modifications, medication changes, or additional support.
Alerts and Notifications: When RPM data deviates from the patient’s baseline or crosses predefined thresholds, healthcare providers receive alerts. These alerts trigger proactive responses and care plan adjustments.
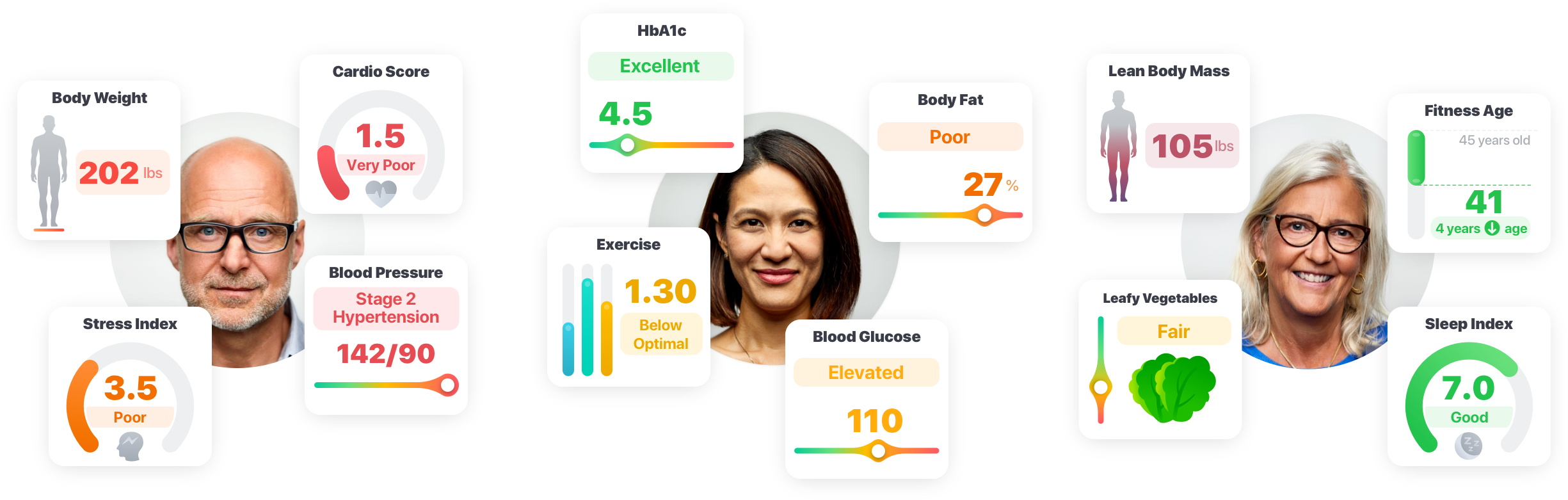
Predictive Analytics in Resource Allocation and Triage within RPM Programs
Predictive analytics plays a pivotal role in the resource allocation and triage of patients in Remote Patient Monitoring (RPM) programs, particularly in scenarios involving a large number of patients. These advanced analytical tools help healthcare providers efficiently distribute their resources, identify high-risk patients, and prioritize interventions.
Risk Stratification: Predictive analytics models assess patient data in near real-time, considering factors like vital signs, historical health records, and lifestyle information. These models assign risk scores to patients, categorizing them based on their likelihood of experiencing health deteriorations. This risk stratification allows providers to distinguish between low-risk and high-risk patients.
Resource Allocation: By understanding the risk level of each patient, healthcare providers can allocate their resources more effectively. High-risk patients, as identified by predictive analytics, require more frequent monitoring, interventions, and support.
Proactive Interventions: Predictive analytics provides insights into potential health issues before they escalate. For patients at high risk of deterioration, healthcare providers can proactively intervene to prevent possibly adverse events. This might involve adjusting medication, suggesting lifestyle changes, or arranging telehealth consultations.
Alert Triage: In RPM programs with a large patient population, automated alerts generated by RPM devices or monitoring platforms can inundate healthcare providers. Predictive analytics helps prioritize these alerts by assessing their clinical significance based on each patient’s risk profile. High-risk patients’ alerts are addressed promptly, ensuring timely responses.
Optimizing Clinical Workflows: Predictive analytics can streamline clinical workflows by presenting relevant patient data to healthcare providers in a prioritized manner. This ensures that clinicians can focus on high-priority cases, reducing administrative burdens and improving patient care.
Preventing Overwhelm: In large-scale RPM programs, healthcare providers might be inundated with data from hundreds or thousands of patients. Predictive analytics filters this data, helping providers manage the patient load effectively.
Resource Efficiency: Resource allocation based on predictive analytics leads to significant efficiency improvements. Healthcare organizations can avoid the overuse of resources for low-risk patients, which can be costly and inefficient. Instead, resources are dedicated to where they’re most needed.
Scalability: Predictive analytics is scalable and adaptable to the size of the patient population. Whether a program monitors a few hundred patients or several thousand, predictive models can effectively categorize patients and support resource allocation.
Continuous Improvement: Predictive analytics helps healthcare providers continually fine-tune their RPM programs. Data from predictive models can be used to refine risk assessment algorithms and optimize care protocols over time.
Cost Savings: By accurately allocating resources and focusing interventions on high-risk patients, healthcare organizations can realize significant cost savings. Preventing hospitalizations and emergency room visits for high-risk patients not only improves patient outcomes but also reduces healthcare costs.
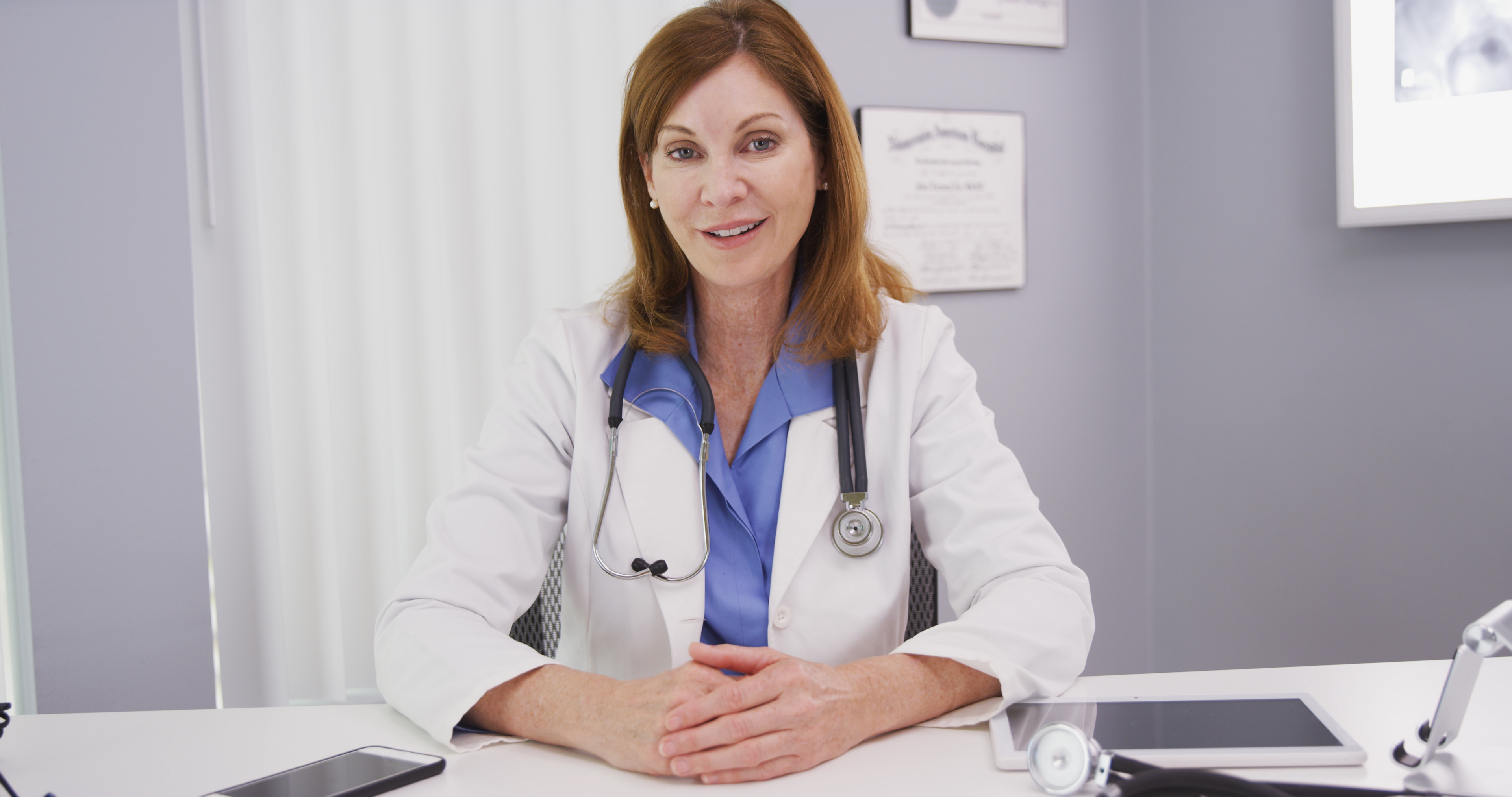
Predictive Analytics in Medication Adherence and Adverse Event Prediction within RPM Programs
Predictive analytics plays a key role in enhancing medication adherence and predicting adverse events within Remote Patient Monitoring (RPM) programs. By leveraging advanced data analysis and machine learning algorithms, healthcare providers can better support patients in following their medication regimens and proactively anticipate potential adverse events.
Medication Adherence
Personalized Adherence Plans: Predictive analytics models can create personalized medication adherence plans for each patient. These plans consider various factors, including the patient’s medical history, lifestyle, and the specific medication they are prescribed. By tailoring the plan to individual needs, patients are more likely to adhere to their medication regimen.
Alerts and Reminders: Predictive models can generate automated alerts and reminders for patients to take their medications. These reminders can be sent through various channels, such as mobile apps, SMS, or email, based on the patient’s preferences. The timing and content of these reminders are optimized to maximize adherence.
Early Non-Adherence Detection: Predictive analytics can identify patients who are at risk of non-adherence before it becomes a significant issue. By analyzing patterns in patient data, such as missed doses or delayed refills, the system can alert healthcare providers to intervene and address potential barriers to adherence.
Behavioral Interventions: Predictive models can predict patient behaviors and barriers to adherence. This information enables healthcare providers to implement targeted behavioral interventions. For example, if a patient is predicted to be at risk of forgetting to take their medication, the provider can offer strategies like setting alarms or using pill organizers.
Outcome Forecasting: Predictive analytics can assess the potential impact of medication non-adherence on a patient’s health outcomes. By quantifying these risks, healthcare providers can educate patients about the importance of adherence and the potential consequences of non-compliance.
Adverse Event Prediction
Early Warning Systems: Predictive analytics can establish early warning systems for adverse events. By analyzing patient data, including vital signs, symptoms, and medication interactions, the system can identify signs of adverse events before they become critical. This enables healthcare providers to intervene promptly.
Drug Interaction Detection: Predictive models can assess the risk of adverse events related to drug interactions. By considering a patient’s medication history and known drug interactions, the system can predict potential issues and alert healthcare providers.
Customized Monitoring: Predictive analytics can recommend customized monitoring plans for patients at higher risk of adverse events. For example, patients with a history of allergies or specific comorbidities may require more frequent monitoring. By tailoring monitoring to individual risk profiles, resources can be allocated more efficiently.
Clinical Decision Support: Predictive analytics offers decision support to healthcare providers. When a potential adverse event is predicted, the system can suggest appropriate interventions or adjustments to the patient’s treatment plan. This empowers providers to take proactive measures to mitigate risks.
Patient Education: Predictive analytics can also inform patient education efforts. Patients can receive information about potential adverse events associated with their medications, helping them recognize warning signs and take preventive actions.
Predictive Analytics in Population Health Management within RPM Programs
Predictive analytics plays a pivotal role in population health management within Remote Patient Monitoring (RPM) programs. By harnessing data-driven insights and advanced modeling techniques, healthcare providers can enhance the care of diverse patient populations.
Preventive Strategies: Predictive models can forecast health trends within a specific population. By identifying potential health threats, healthcare providers can implement preventive strategies, such as vaccination campaigns or health education initiatives. These strategies are designed to mitigate risks, protect vulnerable populations, and reduce the overall burden on the healthcare system.
Chronic Disease Management: Many RPM programs focus on chronic disease management. Predictive analytics can assist in chronic disease management by predicting disease exacerbations, medication adherence issues, or lifestyle factors that may impact disease progression. Providers can then offer targeted interventions, such as telehealth consultations or medication adjustments.
Quality Improvement: Predictive analytics supports ongoing quality improvement efforts. By analyzing patient outcomes and comparing them with predictions, healthcare providers can refine their care strategies. This feedback loop ensures that the population health management program evolves to deliver better care over time.
Patient Engagement: Personalized communication and engagement strategies are essential for population health management. Predictive analytics can recommend the most effective communication channels and engagement methods for different patient segments. This enhances patient participation in monitoring and self-management.
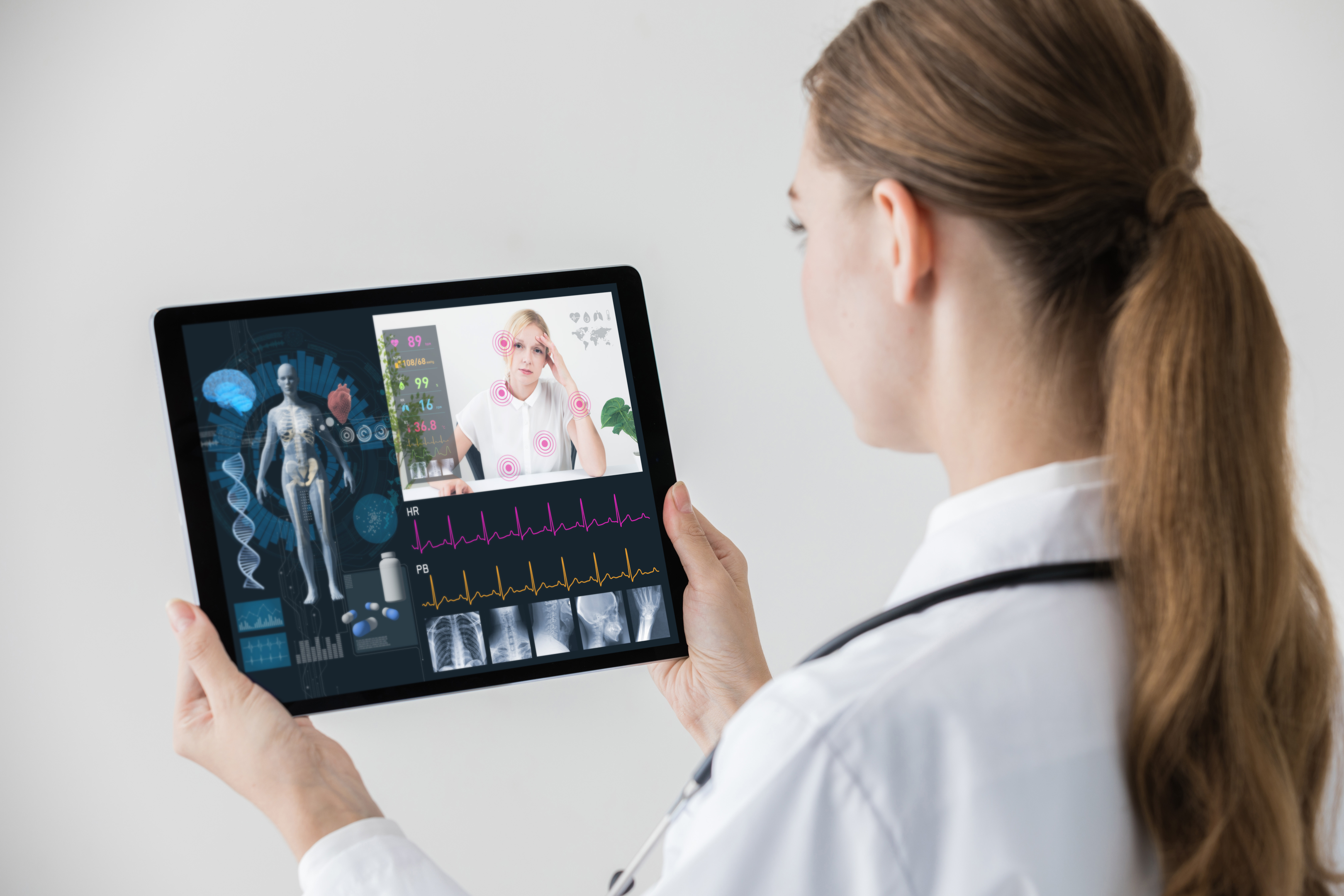
Predictive Analytics Help Reduce Hospital Readmissions within RPM Programs
Predictive analytics plays a critical role in reducing hospital readmissions within Remote Patient Monitoring (RPM) programs. By leveraging data-driven insights and predictive models, healthcare providers can identify patients at high risk of readmission and implement targeted interventions to prevent costly and potentially avoidable return trips to the hospital.
Early Identification of High-Risk Patients: Predictive models can identify patients who are at the highest risk of hospital readmission. These models take into account factors such as chronic conditions, recent hospitalizations, medication adherence, and vital sign trends. By identifying high-risk patients early, healthcare providers can allocate resources and interventions where they are most needed.
Personalized Interventions: Once high-risk patients are identified, personalized interventions can be designed based on the specific factors contributing to their risk. For example, if medication non-adherence is a significant risk factor, interventions may include medication management programs, reminders, or telehealth check-ins to address medication-related issues. These interventions are tailored to each patient’s unique needs.
Proactive Approach: Predictive analytics provides a proactive approach to reducing hospital readmissions. By monitoring patients’ health data in near real-time, providers can detect warning signs of health deterioration or non-adherence to care plans. Timely interventions may involve adjusting medications, coordinating with home health services, or providing additional education and support.
Care Coordination: Collaboration among healthcare providers is crucial in preventing readmissions. Predictive analytics can facilitate care coordination by alerting relevant healthcare teams and providers about high-risk patients. This ensures that everyone involved in a patient’s care is informed and can take appropriate actions to prevent readmissions.
Continuous Improvement: Predictive analytics also supports continuous improvement in care delivery. By analyzing readmission data and intervention outcomes, healthcare providers can refine their predictive models and interventions over time, leading to better results and fewer readmissions.
Elevate Your Patient Care Efforts with HealthSnap’s Industry-Leading RPM Program
HealthSnap’s Virtual Care Management Platform is revolutionizing Remote Patient Monitoring and Chronic Care Management by offering continuous, proactive, and remote patient care programs to high-risk chronic populations. HealthSnap is HIPAA-compliant and HITRUST-certified, ensuring the highest standards of compliance, reliability, and security to support seamless care coordination on a large scale.
To learn more about HealthSnap’s virtual care management programs and to schedule a free demo, call us today at 888-780-1872 (Ext. 3) or click here to set up a consultation with our experts.
Leave A Comment
You must be logged in to post a comment.