The integration of artificial intelligence (AI) into Remote Patient Monitoring (RPM) is revolutionizing healthcare by enhancing patient care, increasing efficiency, and enabling early intervention. RPM leverages technology to monitor patients’ health conditions remotely, reducing the need for frequent in-person visits. The incorporation of AI algorithms further augments the potential of RPM by analyzing vast amounts of patient data to detect trends, anomalies, and potential issues. In this guide, we will explore the top transformative use cases of AI in Remote Patient Monitoring in 2024 and beyond.
AI in RPM Use Case: Early Detection of Health Deterioration
Early detection of health deterioration is a critical aspect of patient care, especially for individuals with chronic conditions or those recovering from acute illnesses. Traditionally, healthcare providers relied on periodic in-person visits to monitor patients’ health status, making it challenging to detect subtle changes in real-time. The integration of artificial intelligence into Remote Patient Monitoring (RPM) is strengthening this process by enabling continuous data collection, analysis, and early identification of potential health issues.
The Role of AI in Early Detection
AI algorithms play a pivotal role in early detection by analyzing vast amounts of patient data collected through wearable devices, sensors, and patient-reported information. This data includes vital signs such as heart rate, blood pressure, respiratory rate, and more. AI models process this data to establish personalized baselines for each patient, accounting for factors like age, gender, medical history, and current health status. Once these baselines are established, the AI system continuously monitors incoming data for deviations from the norm.
Key Components of AI-Enabled Early Detection
-
Near Real-Time Monitoring: Wearable devices and sensors equipped with AI technology continuously gather patient data, ensuring a constant stream of information. This near real-time data collection and monitoring allows AI algorithms to detect even the slightest deviations from established baselines.
-
Pattern Recognition: AI models excel at pattern recognition. By analyzing patterns in the collected data, such as irregular heart rhythms, abrupt spikes or drops in vital signs, and sudden changes in activity levels, AI can identify potential signs of health deterioration that might otherwise go unnoticed.
-
Anomaly Detection: AI algorithms are trained to identify anomalies that fall outside the range of normal variations. When an anomaly is detected, the AI system alerts healthcare providers, enabling them to intervene promptly and initiate appropriate actions.
-
Predictive Analysis: Beyond detecting current anomalies, AI can also predict potential health issues based on historical data trends. For example, if a patient’s heart rate variability has been gradually decreasing over time, AI can alert medical professionals to the increased risk of a cardiac event.
Benefits of Early Detection through AI-Enabled Remote Patient Monitoring
-
Timely Intervention: AI-enabled RPM facilitates early intervention by detecting subtle changes in patients’ health status. This timely response can prevent the progression of health issues and reduce the likelihood of complications.
-
Reduced Hospitalizations: By identifying health deterioration early, AI in healthcare can help reduce the need for hospitalizations. Patients can receive necessary care in their homes, resulting in cost savings for both patients and healthcare systems.
-
Improved Patient Outcomes: Early detection and intervention lead to improved patient outcomes, as potential health crises can be averted, leading to better quality of life for patients.
-
Data-Driven Insights: The continuous collection and analysis of patient data generate valuable insights for medical professionals. These insights can inform treatment plans, medication adjustments, and lifestyle recommendations.
Challenges and Considerations
-
Algorithm Accuracy: AI algorithms must be highly accurate to minimize false positives and false negatives, ensuring reliable early detection.
-
Data Security: Protecting patient data from breaches is paramount. Robust data security measures must be in place to safeguard sensitive health information.
-
User Engagement: Patients need to actively participate in remote monitoring for effective early detection. Ensuring patient engagement and adherence to wearable usage is vital.
AI in Remote Patient Monitoring Use Case: Personalized Treatment Plans
Personalized treatment plans are a cornerstone of effective healthcare, especially in managing chronic conditions and complex medical cases. The integration of artificial intelligence (AI) into RPM is changing the way healthcare professionals create and implement personalized treatment strategies.
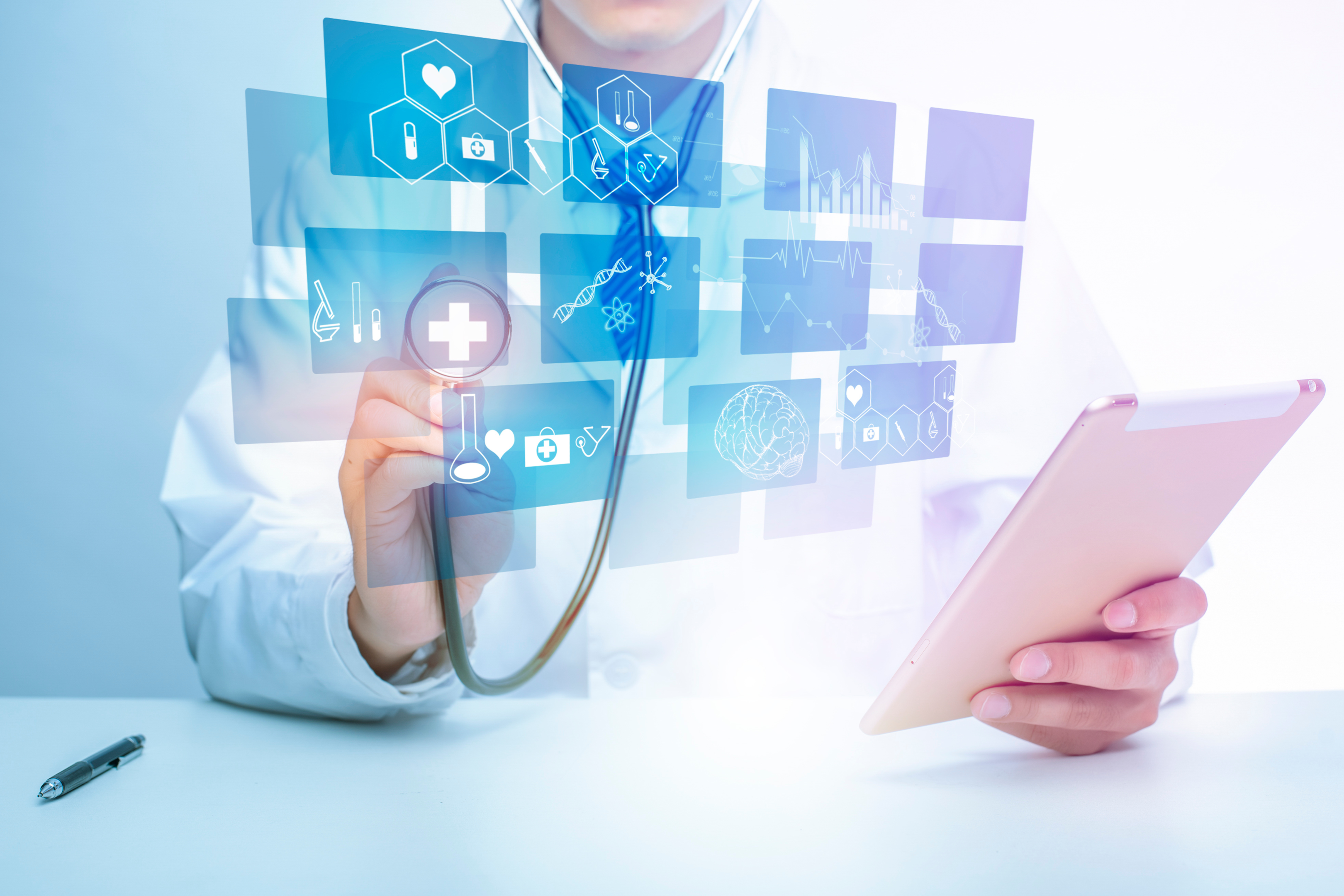
The Role of AI in Personalized Treatment Plans
AI brings a data-driven and holistic approach to tailoring treatment plans for patients. By analyzing vast amounts of patient data, including medical history, genetics, lifestyle choices, and responses to previous treatments, AI algorithms can generate insights that inform the creation of personalized care strategies.
Key Components of AI-Enabled Personalized Treatment Plans
-
Data Integration: AI aggregates data from various sources, such as electronic health records, wearable devices, medical imaging, and patient-reported information. This comprehensive dataset provides a holistic view of the patient’s health status.
-
Predictive Analytics: AI algorithms leverage historical data to predict potential outcomes based on different treatment approaches. This allows healthcare providers to assess the likely effectiveness of various interventions before implementation.
-
Risk Assessment: AI assesses the patient’s risk factors for complications or adverse events. By considering individual characteristics and medical history, AI identifies high-risk patients who require targeted interventions.
-
Treatment Recommendations: Based on data analysis, AI generates personalized treatment recommendations. These recommendations may include medication adjustments, lifestyle modifications, dietary changes, and physical activity guidelines.
Benefits of Personalized Treatment Plans through AI-Enabled RPM
-
Custom Care: AI-driven treatment plans consider each patient’s unique characteristics, optimizing the likelihood of treatment success and patient satisfaction.
-
Optimized Interventions: By analyzing data from similar cases, AI in healthcare can suggest treatment approaches that have proven effective for patients with comparable conditions and profiles.
-
Near Real-Time Adjustments: AI monitors patient progress in near real time, allowing for continuous adjustment of treatment plans based on evolving health data.
-
Patient Empowerment: Personalized treatment plans empower patients to actively participate in their own healthcare by providing insights into how their choices impact their health outcomes.
-
Efficiency and Resource Allocation: AI-optimized treatment plans can lead to more efficient resource allocation, as interventions are targeted and tailored, reducing unnecessary procedures and medications.
Challenges and Considerations
-
Data Quality and Integration: Accurate and comprehensive data are essential for AI to generate meaningful treatment recommendations. Ensuring data accuracy and compatibility across various systems is crucial.
-
Ethical Considerations: Privacy concerns and potential biases in AI algorithms must be addressed to ensure equitable and ethical treatment recommendations.
-
Provider-Patient Relationship: While AI in healthcare enhances treatment planning, maintaining a strong provider-patient relationship remains essential for effective care.
AI in RPM Use Case: Predictive Analytics for High-Risk Patients
Predictive analytics is a powerful tool that combines artificial intelligence and Remote Patient Monitoring to identify patients at high risk of adverse health events. By analyzing patient data and patterns, AI-driven RPM systems can forecast potential health complications and enable healthcare providers to intervene proactively.
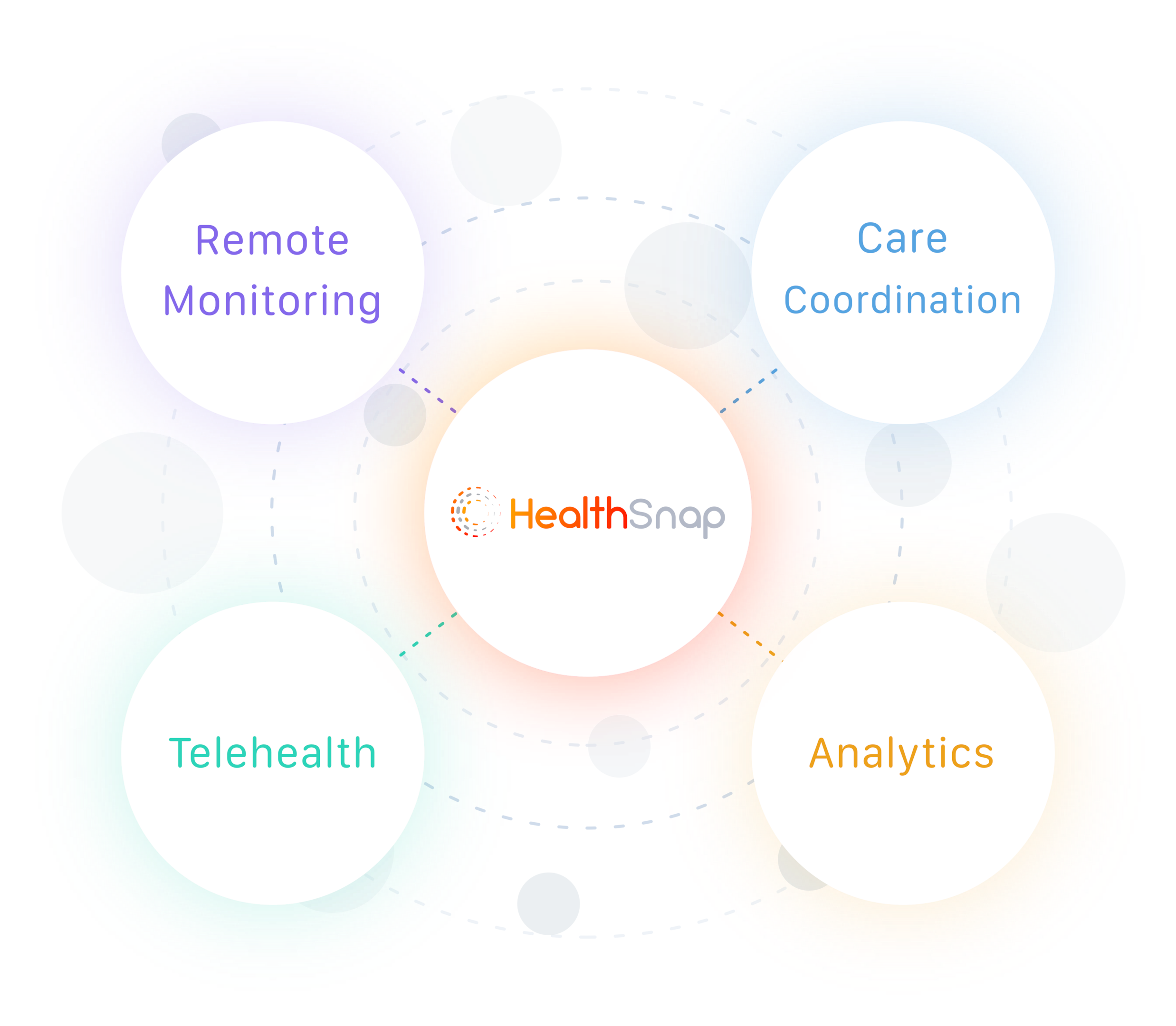
The Role of AI in Predictive Analytics
AI excels at processing large volumes of data and identifying patterns that might go unnoticed by human observation alone. In predictive analytics, AI algorithms analyze historical patient data, real-time health metrics, and other relevant information to forecast future health outcomes. This enables healthcare providers to focus their attention on high-risk patients who require immediate intervention.
Key Components of AI-Enabled Predictive Analytics
-
Data Collection: RPM systems collect a wealth of patient data, including vital signs, lab results, medication adherence, lifestyle habits, and historical health records. This data forms the foundation for predictive analysis.
-
Machine Learning Algorithms: AI employs machine learning algorithms to identify patterns, trends, and correlations within the collected data. These algorithms continuously learn from new data, improving their predictive accuracy over time.
-
Risk Stratification: By analyzing data patterns, AI categorizes patients into risk groups based on the likelihood of adverse events. This stratification helps healthcare providers allocate resources effectively and prioritize interventions.
-
Alerts and Notifications: When AI detects patterns indicative of potential health deterioration, it generates alerts and notifications for healthcare providers. These alerts prompt timely interventions to prevent or mitigate adverse outcomes.
Benefits of Predictive Analytics for High-Risk Patients
-
Early Intervention: AI identifies high-risk patients before symptoms become severe, enabling early intervention and reducing the likelihood of complications.
-
Resource Optimization: Predictive analytics helps healthcare providers allocate resources more efficiently by focusing on patients who need immediate attention, thus reducing healthcare costs.
-
Patient-Centric Care: High-risk patients receive personalized attention and tailored interventions, enhancing the quality of care and improving patient satisfaction.
-
Preventive Measures: Predictive analytics not only identifies current high-risk patients but also forecasts potential risks, allowing providers to implement preventive measures to mitigate adverse events.
-
Data-Driven Decision-Making: Healthcare providers can make informed decisions based on AI-generated insights, leading to improved patient outcomes and reduced hospital readmissions.
Challenges and Considerations
-
Data Quality and Integration: Accurate, complete, and interoperable data sources are necessary for reliable predictive analytics.
-
Algorithm Transparency: AI algorithms must be transparent and understandable to build trust among healthcare providers and patients.
-
Ethical Implications: Ensuring patient privacy, consent, and equitable care is paramount when implementing predictive analytics.
AI in RPM Use Case: Enhanced Medication Adherence
Medication non-adherence is a significant challenge in healthcare, leading to compromised treatment outcomes and increased healthcare costs. The integration of artificial intelligence (AI) into Remote Patient Monitoring (RPM) offers a transformative solution by improving medication adherence through personalized interventions and continuous monitoring.
The Role of AI in Enhancing Medication Adherence
AI technology plays a vital role in promoting medication adherence by providing personalized support, reminders, and insights to patients. Through RPM, AI monitors patient behavior and responses to treatment, identifies adherence patterns, and predicts potential non-adherence issues.
Key Components of AI-Enabled Enhanced Medication Adherence
-
Behavioral Analysis: AI algorithms analyze patient behavior and engagement patterns to predict when a patient might forget or skip medication doses.
-
Personalized Reminders: AI sends personalized reminders and notifications to patients, ensuring they take their medications as prescribed. These reminders can be tailored to patients’ preferences and schedules.
-
Data Integration: AI integrates with electronic health records and wearable devices to track medication adherence and provide real-time feedback to both patients and healthcare providers.
-
Predictive Insights: AI predicts adherence challenges based on historical and real-time data, allowing healthcare providers to intervene before non-adherence becomes a significant issue.
-
Patient Engagement: AI engages patients by providing educational content, explaining the importance of medication adherence, and addressing concerns or misconceptions.
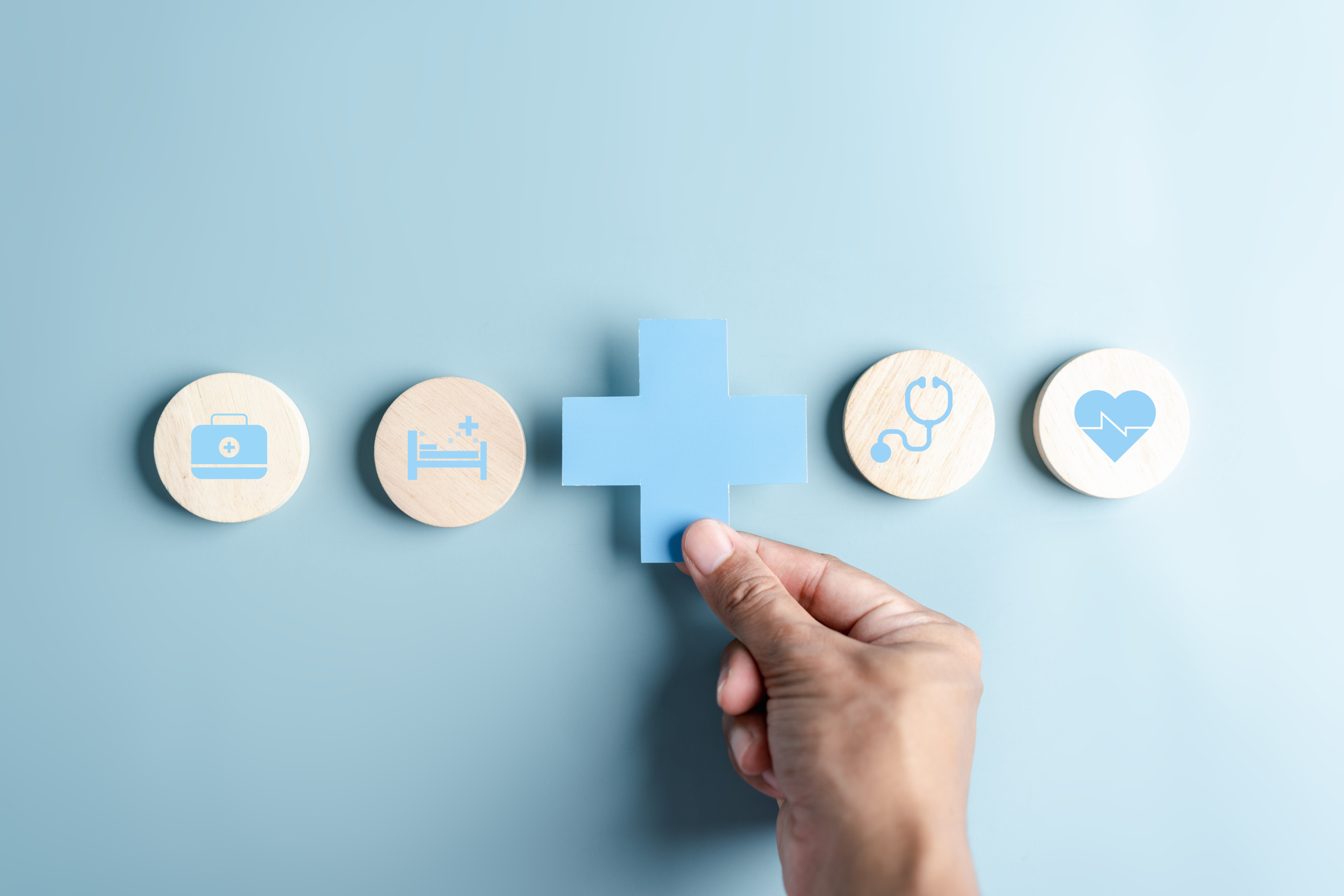
Benefits of AI-Enabled Enhanced Medication Adherence
-
Improved Adherence: Personalized reminders and real-time feedback from AI encourage patients to adhere to their medication regimens, leading to better treatment outcomes.
-
Reduced Health Risks: Consistent medication adherence helps manage chronic conditions and reduces the risk of complications or disease progression.
-
Cost Savings: Improved medication adherence can lead to fewer hospitalizations, emergency room visits, and disease-related complications, resulting in cost savings for both patients and healthcare systems.
-
Patient Empowerment: AI empowers patients to take an active role in their health management by providing them with tools and resources to stay on track with their medications.
-
Data-Driven Insights: Healthcare providers gain insights into patients’ adherence patterns and responses to interventions, enabling them to tailor strategies and interventions more effectively.
Challenges and Considerations
-
Patient Privacy: AI systems must handle patient data securely and comply with privacy regulations to protect sensitive health information.
-
User Acceptance: Patients’ comfort and willingness to engage with AI-driven reminders and interventions are critical for successful implementation.
-
Cultural and Language Considerations: AI must be sensitive to cultural differences and language preferences to effectively engage diverse patient populations.
Generative AI and Healthcare Use Cases
Generative AI (Gen AI) such as ChatGPT, emerges as a powerful tool capable of changing the course of healthcare for the better. Employing deep-learning algorithms, Gen-AI technology crafts fresh content spanning text, audio, code, and beyond.
Generative AI confronts unstructured datasets—data lacking predetermined structure—which typically pose challenges in analysis. These datasets prove a potential game-changer for healthcare operations, housing unstructured information like clinical notes, diagnostic images, and medical charts. These datasets, whether in isolation or combined with structured data like insurance claims, offer avenues for innovation.
Healthcare professionals and technologists witness the emergence of multiple applications for Gen AI in healthcare, evoking both excitement and caution. Despite AI’s longstanding use in healthcare—examples include predictive adverse-event analysis and optimization of operating-room schedules—Gen AI tools such as ChatGPT introduce a fresh dimension. It holds the key to unlocking a portion of the industry’s untapped $1 trillion potential by automating monotonous tasks, granting instant access to years of clinical data, and rejuvenating healthcare systems.
Actualizing this potential necessitates the integration of these models into existing analytics and AI roadmaps, alongside meticulous risk assessment. The sensitive nature of patient healthcare data calls for rigorous data security measures. Given Gen AI’s potential for incorrect responses, human involvement (“human in the loop”) remains crucial to ensure patient benefit. As regulatory and legal structures crystallize, user safety takes precedence.
Notwithstanding the precautions inherent in adopting Gen AI in healthcare, the potential is too vast for organizations to overlook. Here’s how private payers and healthcare providers can embark on this impactful journey.
Harnessing Gen AI across Private Payers, Hospitals, and Physician Groups
In the short term, Gen-AI technology offers a multitude of possibilities for insurance executives, hospital administrators, and physician group operators across the value chain. The applications span from maintaining continuity of care to improving medical diagnosis to deciphering network and market insights to efficient medical records management to embracing value-based care for better health outcomes.
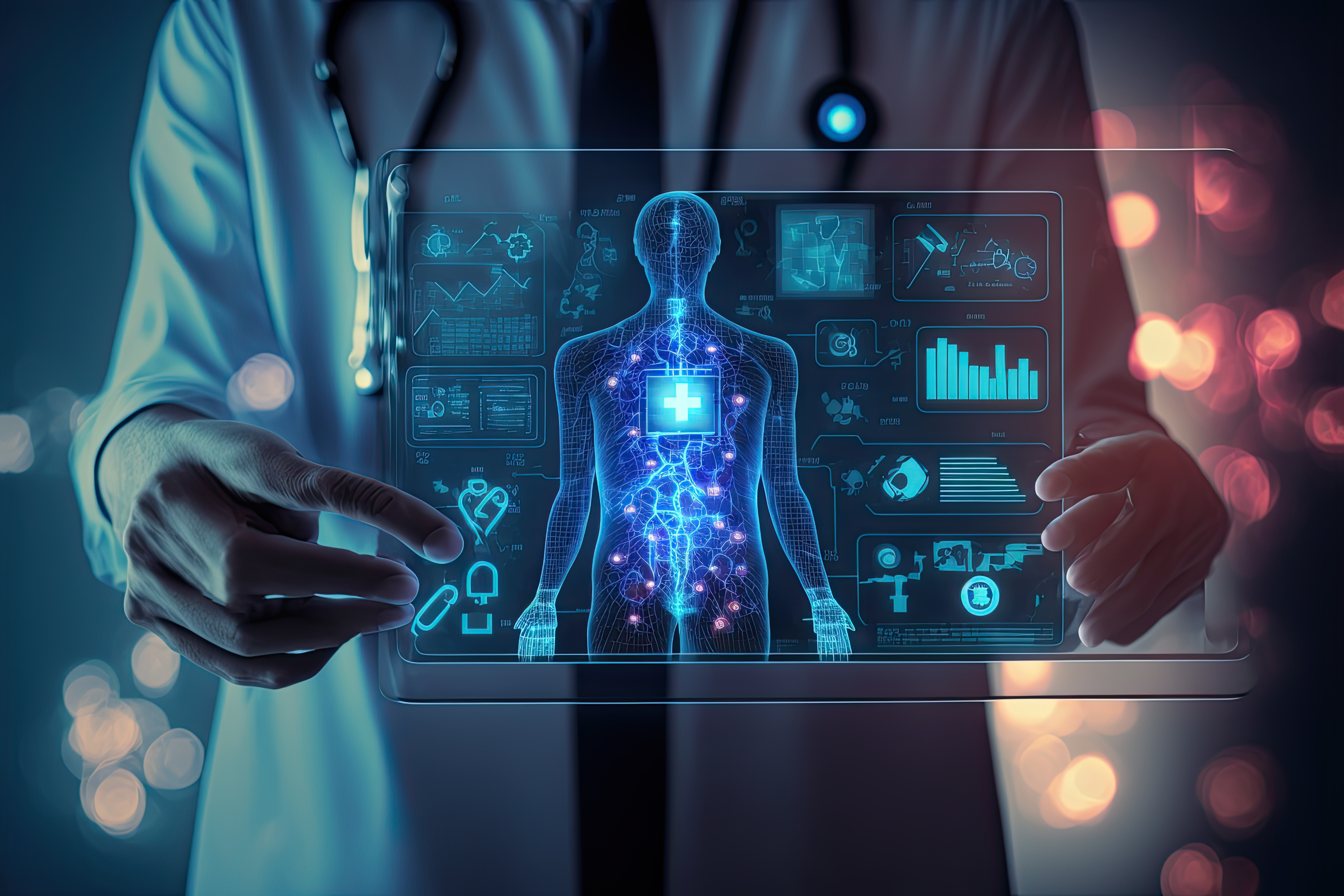
Private Payers
Private payers grapple with the demands for personalized and convenient healthcare services, amidst mounting competitive pressures and escalating healthcare expenses. Gen AI offers a remedy, enhancing operational efficiency while elevating patient and customer service quality.
While certain operations necessitate human intervention, Gen-AI technology supplements various processes. Core administrative functions and interactions with members and providers entail sifting through voluminous logs and data—a time-consuming task. Gen AI swiftly and automatically summarizes this data, freeing up resources for more intricate needs.
Gen AI holds immense potential to improve member services. Customized coverage summaries and information extraction from diverse plan types become seamless tasks. Speeding up and enhancing resolution of claims denials is another forte of Gen AI. It concisely summarizes denial letters, pinpoints relevant codes, highlights denial reasons, and suggests subsequent actions. At the same time, human oversight remains integral.
The technology’s capabilities extend to streamlining health insurance prior authorization and claims processing, reducing time-consuming efforts. These products convert unstructured data into structured formats, enabling real-time benefits verification, including precise calculations of out-of-pocket expenses.
Hospitals and Physician Groups
Within hospital and physician group settings, Gen-AI’s impact spans continuity of care, clinical operations, contracting, and corporate functions.
Corporate functions within hospitals, often operating in isolated silos, stand to benefit from Gen AI’s prowess. It taps into unstructured purchase and accounts payable data, leveraging gen-AI chatbots to address common IT and HR queries. Enhanced employee experiences and reduced administrative costs are within reach.
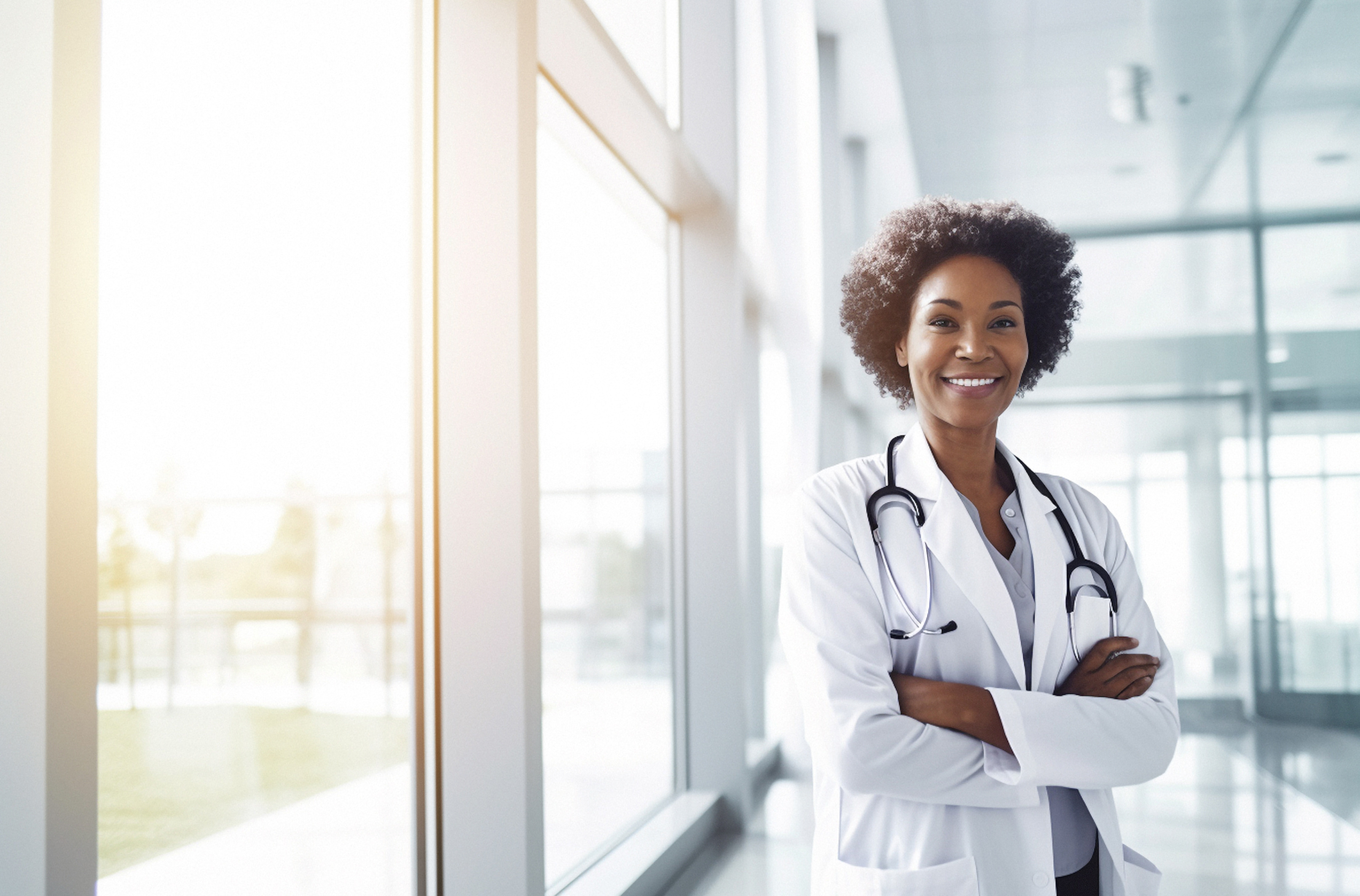
Gen AI brings efficiency to clinical operations, relieving providers and staff of time-consuming tasks such as form-filling and note-taking. Physician groups and hospital providers face numerous administrative burdens, contributing to burnout. Gen AI can generate discharge summaries, instructions, and real-time checklists, easing the load.
The interface with electronic health records (EHRs) also sees a transformation. Gen AI’s language synthesis capability augments EHR functionality, aiding providers in accessing and updating patient information accurately. Pilot programs already integrate Gen AI to streamline operations, prepopulate visit summaries, and offer decision support.
Human involvement remains pivotal in Gen AI-driven processes, balancing technological prowess with human expertise. Robust risk and compliance review remains essential for synthesized content.
Navigating the Future of Healthcare with Generative AI
The convergence of Gen AI with healthcare holds immense promise, demanding strategic evaluation and responsible implementation. Healthcare industry leaders and medical professionals must set out on a journey of change, guided by the following principles.
Assess the Landscape
Determining Gen AI’s applications requires cross-functional collaboration to identify potential value within divisions. Avoiding ad hoc approaches, integrating prioritized use cases into the AI roadmap ensures effective implementation.
Analyze Data
To extract maximum value, enrich data quality through strategic partnerships within the healthcare industry as well as interoperability investments. Strengthening the AI tech stack is paramount for leveraging large language models.
Address Risks and Bias
Mitigating risks, particularly medical data privacy and bias concerns, necessitates comprehensive frameworks. These medical care frameworks should encompass data security, bias fairness, regulatory compliance, and accountability.
Invest in People and Partnerships
The evolution introduced by Gen AI impacts roles and workflows, requiring a human-in-the-loop approach. Training, guidelines, and user-friendly interfaces are essential to ensure successful integration. Strategic partnerships with technology firms can expedite implementation while adhering to regulatory standards.
Gen AI holds great potential that extends beyond imagination. By championing responsible adoption, health care leaders can reshape the industry, delivering enhanced experiences, equitable outcomes, and unparalleled efficiency. The journey begins now.
HealthSnap: Impacting Chronic Disease Outcomes Through Virtual Care Management Solutions
HealthSnap is leading the way in pioneering virtual care management solutions, making a tangible impact on chronic disease management. Our advanced Remote Patient Monitoring (RPM) program is tailored for healthcare organizations, centering on cutting-edge cellular-enabled RPM devices. Our Virtual Care Management Platform seamlessly interfaces with over 80 Electronic Health Record (EHR) systems.
For healthcare providers seeking comprehensive solutions, HealthSnap offers an innovative, integrated virtual care management platform. Our full-service options encompass patient care management, ideal for those without in-house virtual care capabilities. To explore the potential of our Remote Patient Monitoring and Chronic Care Management platform and services, contact us at 888-780-1872 (Ext. 3) for a complimentary demo or click here to schedule a consultation.
Leave A Comment
You must be logged in to post a comment.